Initially, credit scoring was used mainly as a tool for underwriting retail credit, such as residential mortgages, credit cards, installment loans, and small business credits. In current times, credit scoring is used to administer default risk across the entire credit portfolio of a financial institution. It covers firms, sovereigns, local authorities, project finance, and financial institutions. The credit scoring models are no longer used only for credit approval, but also in other contexts such as pricing, provisioning, regulatory and economic capital calculation, and securitization.
Application scoring is the evaluation of the risk of the borrower when the credit is being granted. In the banking business, it is vital to monitor the risk of approved transactions in the banking book and observe the status of the approved loans during their lifetime. When a loan agreement is breached, collection scoring supports decisions and updates the risk parameters. An overview of scoring techniques through the various customer stages is seen in the figure below.
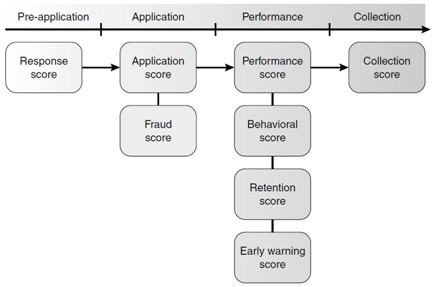
Figure: Overview of various scoring methods during a loan process
Marketing score
Before a loan is applied, marketing campaigns need to address potential customers. Heavy amount of competition between various financial institutions that offer similar products has led to change the market from a supply market to a demand market. The bargaining power is in the hands of the consumer as he/she decides which offer to obtain. As the markets are saturated with similar products and services, there is a growing need to analyse which financial product a customer is most likely to accept. This consumer behavior and decision would result in the profit of the financial institution.
Marketing is an essential part of an institution’s strategy and success. The bigger the market share is of the company, the larger the assets and income are. Successful marketing aims to target the “right” product to the “right” customer with its media campaigns. Response scoring is based on the probability that a potential customer will react to a marketing campaign such as,
- a direct mailing for a new product
- TV/radio advertisement
- billboard advertisements
- referral promotions.
Acceptance scoring goes on to assesses the likelihood of a customer accepting a variant of a financial product or an offer. The thumb rule of marketing are used just the same in a marketing score i.e. the four Ps marketing mix:
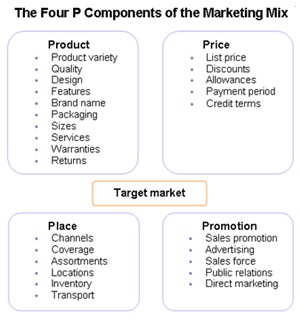
- Product: the offered services need to correspond with the prospective customer’s needs.
- Pricing: the price needs to be set such that it is affordable for the consumer and also covers the cost of manufacturing, logistics, and promotions. Many pricing activities take place to attract attention towards the product. This includes promotions (buy one get one free, discounts, rebates etc.
- Promotion: These are activities carried out to promote awareness and sales of the product. Examples of promotions are advertising, publicity, personal selling, brand, product and company recognition.
- Place: This pertains to the channel through which the banking products or services are sold. For instance, density of a retail network, internet-banking, specificity of the customer group. The place also refers to placement, the “physical” distribution to the customer.
Other variables that matter in marketing are satisfaction levels of the consumers, relationships with customers, and the competition. Going further, the marketing variables can be augmented with variables for repurchase behavior (recency, frequency and monetary), and risk management variables. In order to cross-sell applications, the internal knowledge of the customer resulting from the risk management databases gives the manager useful insight on targeting creditworthy customers.
The aim of the marketing score is to reduce the cost of customer acquisition and to minimize the customer inconvenience and dissonance. The marketing activities are targeted at a set number of people with good profit potential that receive a score above the threshold. These people are the prospective, potentially new customers and/or existing customers.
Application score
Application scoring systems are used to compile and consolidate all applicant information into one overall score calculating the creditworthiness of loan applicants. The application scores are main inputs used in determining whether a new credit should be approved. This score is the initial score given to the applicant based on the information available at the time of application. The score gives the probability of repayment problems. High scores represent the high creditworthiness of customers, and therefore they are a safe investment. Contrarily, low scores indicate questionable payers that should be declined credit. An example of an application scorecard is given in the table on page 36. As stated before, a customer is granted a loan when there is a good score that exceeds the cut-off or threshold value. The cut-off levels defines the risk that the bank wants to take and it depends on a bank’s strategy, risk capacity and pricing.
Many a times, there are grey areas of the score range where the approval outcome results from an additional human expert judgment. This is represented in the following figure.
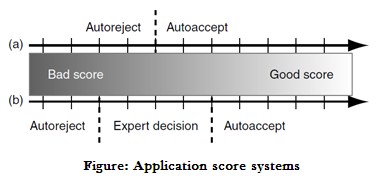
In the above figure, (a) means that in a fully automated application score system, the application is granted credit if the score surpasses the cut-off. The next line (b) shows that in a semi-automated system, the application is automatically approved or declined for high and low scores. For the transitional scores, the decision is aided by ‘Expert decision’ through human investigation.
The score that is allocated further influences the price and amount of the loan can be granted. In a risk-based pricing environment, the price of the loan depends on the risk of the customer. A high-risk customer will be allowed a lower amount to reduce concentration risk on low-quality borrowers. An applicant with scores below the cut-off indicates a high risk and is declined.
Benefits of using an automated application scorecard
- The time to process a new application is reduced multifold. The applications can be examined and scored in real-time, and this serves as a huge competitive advantage for the lending company amidst the existence of the internet and e-commerce.
- There is a consistency is the decisions that are made. This is possible because of an enterprise-wide credit approval policy based on the scorecard, rather than relying on an ad-hoc subjective evaluation of a credit expert.
- By using the scorecard, targeted questionnaires are used during the application process. This prevents the customer from having to fill in the entire application form with unrelated details.
Legal and ethical issues are involved in approving credit. According to The Equal Credit Opportunities Act (1976) and regulation B in the United States, it is prohibited to use characteristics such as gender, marital status, race, whether an applicant receives welfare payments, color, religion, national origin and age in reaching the credit decision. Similarly, when an applicant has been rejected credit, he/she must be given the specific reasons for the decision.
Fraud score
Fraud scoring is based on untrue or illegal information or actions carried out by the customer. This scoring is presented in a variety of ways. A few examples are listed below:
- Application fraud scoring – In this, one measures the probability of the credit applicant providing fraudulent information at the time of application.
- Claim fraud scoring- Here, the assessor ranks orders insurance claims based on the probability of being fraudulent.
- Credit card fraud scoring – This aims at detecting fraudulent credit card transactions.
Fraud scores need to be frequently updated to adapt themselves timely to new fraud mechanisms.
In credit scoring, a manager can easily determine which accounts turn faulty by observing and counting the number of days in payment arrears. In fraud scoring, it is less evident to categorize an insurance claim as fraudulent or a credit application as containing fraudulent information. Unlike other scoring mechanisms, fraud scores are generally difficult to measure ex post what constitutes a fraudulent transaction.
Performance score
Performance scoring is used to ascertain the risk of an existing customer during its performance stage. The performance score often uses a fixed time horizon in the range of one to several years. This period is called the performance period. Because performance scoring is applied on customers, more detailed information is available publicly on a longer time history than for brand new applicants. The figure below shows the process of the application, behavioural, and profit scoring through the assessment period.
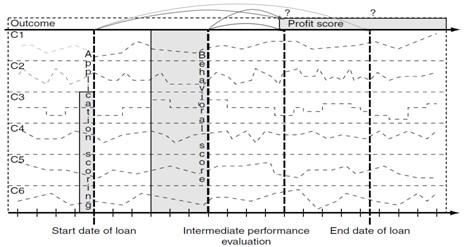
Figure: Application, behavioural, and profit scoring
In the above figure, it is seen that,
- Application scoring takes a snapshot of the counterpart at the beginning of the loan to predict the outcome at the end of the loan.
- Behavioural scoring looks at the recent evolution and behavior of the counterparty to predict its evolution on a shorter time horizon. The counterpart being a customer, more information or characteristics (C) are available on a longer time horizon.
- Profit scoring calculates the risk along a much lengthier time frame. The outcome is measured on a time interval i.e. it is evaluated several times during the customer relationship using increasingly more data.
The main aim of the performance scoring is to study the existing portfolio, its future performance, and losses. The outcomes from this analysis are used for provisioning, regulatory and economic capital calculations. Customers that are high risk are detected and put on a watch-list before they possibly default. Losses can be minimized by active risk measurement such as customer assistance, debt restructuring, and reducing credit limits of revolving credits and taking collateral. The Basel II capital accord lays high emphasis on internal performance scoring systems to monitor the risk with a default prediction horizon of 12 months.
Unsuccessful companies have been termed as “financial failure”, “business failure”, and “bankruptcy.” These are often used interchangeably although their meaning may formally differ. A commonality is that these businesses cannot pay liabilities that have become due, and that results in the discontinuity of their operations. The common assumption underlying these models is that key macroeconomic indicators together with firm characteristics should reflect in the business’ financial statements. The future financial status can be predicted by using the information originating from these statements and complex credit scoring processes. A popular bankruptcy prediction model is Altman’s z-model. Using a sample of 66 manufacturing firms, a linear discriminant using five financial ratios is constructed as follows:
z = 0.012×1 + 0.014×2 + 0.033×3 + 0.006×4 + 0.999×5
where,
x1 = working capital/total assets,
x2 = retained earnings/total assets,
x3 = earnings before interest and taxes/total assets,
x4 = market value equity/book value of total debt,
x5 = sales/total assets.
A high z-score presents a more financially stable firm. For instance, a z-score > 2.99 indicates that the company is situated in the safe zone. A z-score between 1.8 and 2.99 show that that the firm is in a grey zone. Finally, a z-score lower than 1.80 means that the firm is in the distress zone. The z-score model after 1968 later extended itself and refined in various ways.
For retail customers, there is a difference between the information available for new applications versus existing customers. Such information is highly predictive and improves the discrimination quality of the score. For larger counterparts like companies, banks and sovereigns, the difference between application and performance scores is more imprecise. Official financial statements and accounts for companies are available through financial reports, they have a more active debt management and apply more regularly for new loans. The main differences between application and performance scoring are the prediction horizon, lack of a customer relation, and the quality of the customer information.
Behavioural score
Behavioural scoring studies the risk of existing customers laying emphasis on their recently observed behaviour. In this, an assessor forecasts the default risk of the borrower within one year. Application scorecards produce scores measuring the risk of default at the start of the loan (if all application characteristics available). After credit has been approved, institutions can commence monitoring the repayment, and financial behaviour of the customers. This means that the risk of default can be reconsidered using all the recent customer behaviour that can be observed. These are,
- checking account balance,
- changes in employment status,
- missed payments,
- new bureau information
All this behavioural data is compiled into a behavioral score that indicates the likelihood that an existing customer will default. Application scoring takes two snapshots of the customer at different points in time: (i) beginning of the loan and (ii) the default observation point. Behavioural scoring considers the behaviour of the customer during the observation period.
The duration considered for the analysis of performance is generally 1 to 2 years. The observation period is typically between 6 months to 2 years depending on the volatility of the borrower’s behaviour. During this period, variables (checking account balance, employment status, bureau score, number of credits) may be consolidated using different variables e.g.,
- maximum, minimum, average, relative trend, absolute trend,
- most recent value,
- yielding derived variables such as minimum checking account,
- relative trend in bureau score,
- average utilization during last 6 months,
- maximum number of previously missed payments,
- most recent income
When summarizing trends in variables, a manager should know the seasonality effects that may exist, e.g., account balance towards 31st December, may be different from that towards 30th April because of end-of-year effects (bonuses, holiday period). This way, many more variables become available compared to an application scoring context, and input selection becomes necessary.
Behavioural scores are most pertinent to retail customers and have proven to be very useful for credit limit management, provisioning, capital calculations. They are also help the data collection for marketing scores as discussed in previous sections. The behavioral scores are recalculated at frequent time intervals such as weekly, monthly etc. in to understand the changing dynamics in a customer’s behaviour. The figure below provides an example of a behavioral scorecard in combination with application scores.
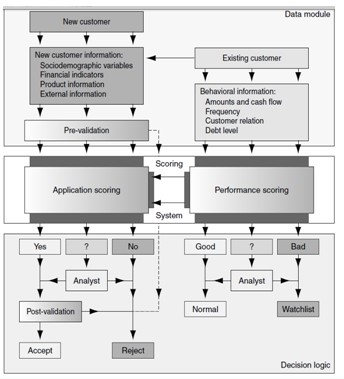
The first score is assigned by the application scorecard. When enough customer data is available, the behavioral scorecard is applied in order to monitor the existing customer’s portfolio, and to improve other scores.