A good-quality portfolio of performing assets leads to a strong credit risk management. The price that is set on loans includes the risk at hand. A diligent selection of assets in a portfolio aims to reduce the chances and impact of high losses. As a part of credit risk management, credit scoring is a practice that evaluates the borrower’s risk and credibility. At the end of this process, a “credit score” is assigned to each customer to indicate its/his/her risk level. A good credit scoring structure need to be highly objective and unbiased.
- high scores indicate minimal risk
- low scores indicate a high probability of risk
The more discriminative a scoring system is, the better the customers are assessed. In the initial stages, risk measures are assigned to every score (score bucket). To verify the quality of the credit scores risk ranking and calibration, ex-post analysis is observed for credit losses per score. Credit scores are commonly categorized into homogeneous groups. These segmented scores are discrete risk estimates that are also known as risk classes and ratings.
In previous times, credit scoring focused on calculating the risk of default by a customer in fulfilling his/her financial obligations, and leading to payment arrears. In recent times, credit scoring has also included loss and exposure risk. Scoring techniques are now important through the whole course of the credit life cycle as it serves as a decision support tool or automated decision algorithm for large customer bases. With growing competition, technological sales advancements, and banking regulations, there has been am increase in the demand for the application of automated and semi-automated scoring systems.
Credit scoring techniques have been executed using different yet related settings. For instance, a credit approval decision is made using solely a judgmental approach by simply studying the details on the application form of the applicant.
- Character: measures the borrower’s character and integrity (reputation, honesty)
- Capital: measures the difference between the borrower’s assets (car, house) and liabilities (renting expenses)
- Collateral: measures the collateral provided in case payment problems occur (house, car)
- Capacity: measures the borrower’s ability to pay (job status, income)
- Condition: measures the borrower’s circumstances (market conditions, competitive pressure, and seasonal character).
This expert-based approach towards credit scoring is used in credit portfolios where limited information and data is available.
An application scorecard was developed using advance statistical and machine-learning techniques that apply a wide range of explanatory variables or characteristics. An application scorecard allocates subscores to each value of these characteristics and the subscores are measured based on the relationship between the characteristic value and the default behavior. All these values are aggregated into one overall application score indicating the total default risk posed by the customer. An example of an application scorecard is given in Table below.
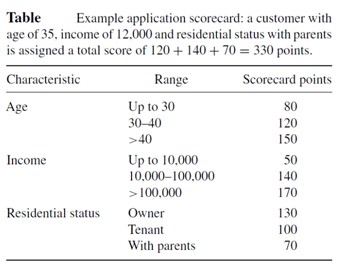
For instance, a new applicant is of age 35, income 12,000, and residential status with parents. With the above scorecard, he will get appointed 330 points which will be compared against a cut-off. Based on this comparison, a credit decision is made. For example, when assuming a cut-off of 300 (400), the above loan will be approved (rejected). It becomes difficult to label a customer as credible or not when the score of a customer is close to the cut-off. For this reason, there is a grey zone around the cut-off and this is investigated upon for customers falling into this region.